This
post was originally published on
this siteIndustries like automotive, robotics, and finance are increasingly implementing computational workloads like simulations, machine learning (ML) model training, and big data analytics to improve their products. For example, automakers rely on simulations to test autonomous driving features, robotics companies train ML algorithms to enhance robot perception capabilities, and financial firms run in-depth analyses to better manage risk, process transactions, and detect fraud.
Some of these workloads, including simulations, are especially complicated to run due to their diversity of components and intensive computational requirements. A driving simulation, for instance, involves generating 3D virtual environments, vehicle sensor data, vehicle dynamics controlling car behavior, and more. A robotics simulation might test hundreds of autonomous delivery robots interacting with each other and other systems in a massive warehouse environment.
AWS Batch is a fully managed service that can help you run batch workloads across a range of AWS compute offerings, including Amazon Elastic Container Service (Amazon ECS), Amazon Elastic Kubernetes Service (Amazon EKS), AWS Fargate, and Amazon EC2 Spot or On-Demand Instances. Traditionally, AWS Batch only allowed single-container jobs and required extra steps to merge all components into a monolithic container. It also did not allow using separate “sidecar” containers, which are auxiliary containers that complement the main application by providing additional services like data logging. This additional effort required coordination across multiple teams, such as software development, IT operations, and quality assurance (QA), because any code change meant rebuilding the entire container.
Now, AWS Batch offers multi-container jobs, making it easier and faster to run large-scale simulations in areas like autonomous vehicles and robotics. These workloads are usually divided between the simulation itself and the system under test (also known as an agent) that interacts with the simulation. These two components are often developed and optimized by different teams. With the ability to run multiple containers per job, you get the advanced scaling, scheduling, and cost optimization offered by AWS Batch, and you can use modular containers representing different components like 3D environments, robot sensors, or monitoring sidecars. In fact, customers such as IPG Automotive, MORAI, and Robotec.ai are already using AWS Batch multi-container jobs to run their simulation software in the cloud.
Let’s see how this works in practice using a simplified example and have some fun trying to solve a maze.
Building a Simulation Running on Containers
In production, you will probably use existing simulation software. For this post, I built a simplified version of an agent/model simulation. If you’re not interested in code details, you can skip this section and go straight to how to configure AWS Batch.
For this simulation, the world to explore is a randomly generated 2D maze. The agent has the task to explore the maze to find a key and then reach the exit. In a way, it is a classic example of pathfinding problems with three locations.
Here’s a sample map of a maze where I highlighted the start (S), end (E), and key (K) locations.
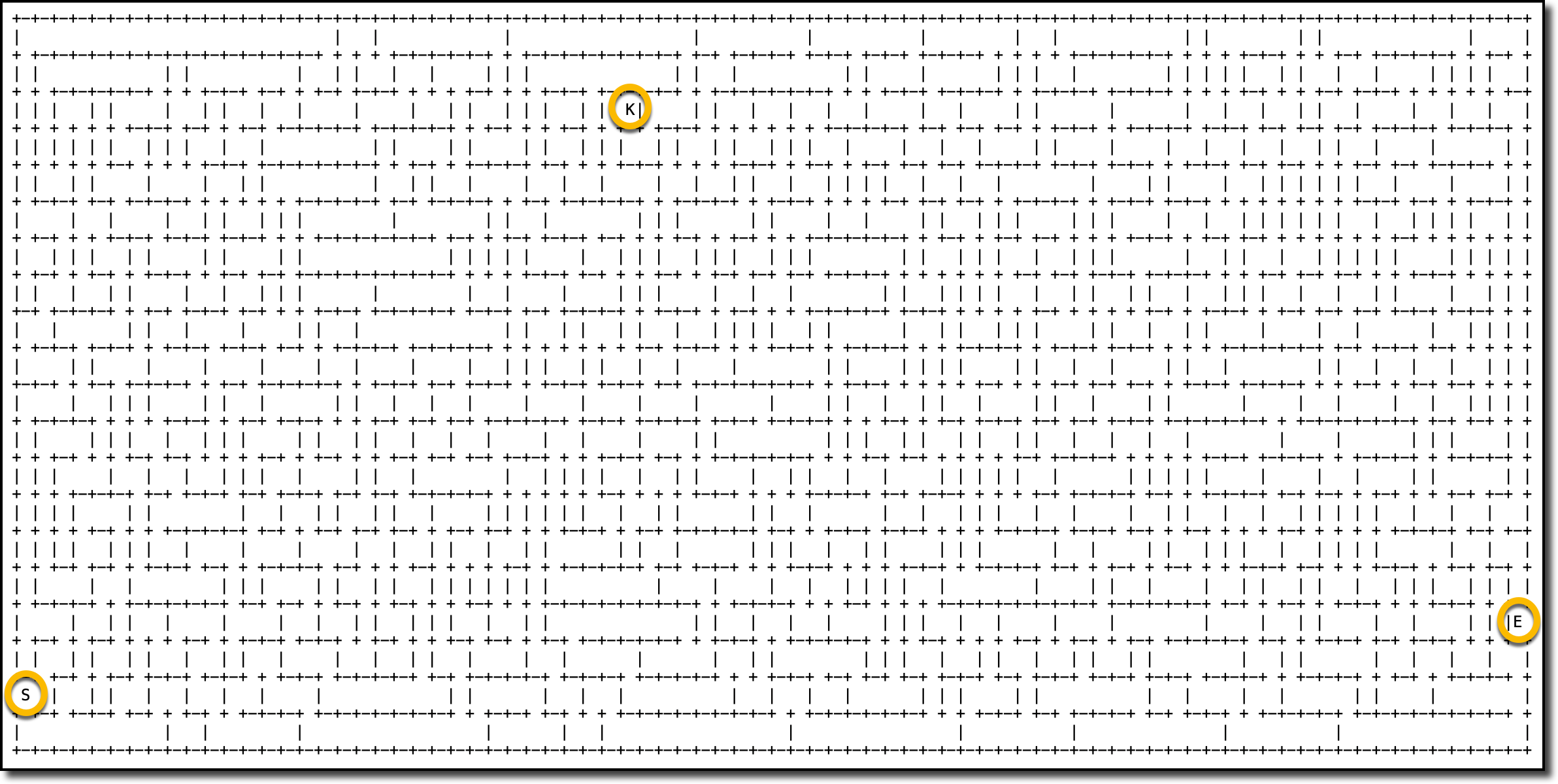
The separation of agent and model into two separate containers allows different teams to work on each of them separately. Each team can focus on improving their own part, for example, to add details to the simulation or to find better strategies for how the agent explores the maze.
Here’s the code of the maze model (app.py
). I used Python for both examples. The model exposes a REST API that the agent can use to move around the maze and know if it has found the key and reached the exit. The maze model uses Flask for the REST API.
import json
import random
from flask import Flask, request, Response
ready = False
# How map data is stored inside a maze
# with size (width x height) = (4 x 3)
#
# 012345678
# 0: +-+-+ +-+
# 1: | | | |
# 2: +-+ +-+-+
# 3: | | | |
# 4: +-+-+ +-+
# 5: | | | | |
# 6: +-+-+-+-+
# 7: Not used
class WrongDirection(Exception):
pass
class Maze:
UP, RIGHT, DOWN, LEFT = 0, 1, 2, 3
OPEN, WALL = 0, 1
@staticmethod
def distance(p1, p2):
(x1, y1) = p1
(x2, y2) = p2
return abs(y2-y1) + abs(x2-x1)
@staticmethod
def random_dir():
return random.randrange(4)
@staticmethod
def go_dir(x, y, d):
if d == Maze.UP:
return (x, y - 1)
elif d == Maze.RIGHT:
return (x + 1, y)
elif d == Maze.DOWN:
return (x, y + 1)
elif d == Maze.LEFT:
return (x - 1, y)
else:
raise WrongDirection(f"Direction: {d}")
def __init__(self, width, height):
self.width = width
self.height = height
self.generate()
def area(self):
return self.width * self.height
def min_lenght(self):
return self.area() / 5
def min_distance(self):
return (self.width + self.height) / 5
def get_pos_dir(self, x, y, d):
if d == Maze.UP:
return self.maze[y][2 * x + 1]
elif d == Maze.RIGHT:
return self.maze[y][2 * x + 2]
elif d == Maze.DOWN:
return self.maze[y + 1][2 * x + 1]
elif d == Maze.LEFT:
return self.maze[y][2 * x]
else:
raise WrongDirection(f"Direction: {d}")
def set_pos_dir(self, x, y, d, v):
if d == Maze.UP:
self.maze[y][2 * x + 1] = v
elif d == Maze.RIGHT:
self.maze[y][2 * x + 2] = v
elif d == Maze.DOWN:
self.maze[y + 1][2 * x + 1] = v
elif d == Maze.LEFT:
self.maze[y][2 * x] = v
else:
WrongDirection(f"Direction: {d} Value: {v}")
def is_inside(self, x, y):
return 0 <= y < self.height and 0 <= x < self.width
def generate(self):
self.maze = []
# Close all borders
for y in range(0, self.height + 1):
self.maze.append([Maze.WALL] * (2 * self.width + 1))
# Get a random starting point on one of the borders
if random.random() < 0.5:
sx = random.randrange(self.width)
if random.random() < 0.5:
sy = 0
self.set_pos_dir(sx, sy, Maze.UP, Maze.OPEN)
else:
sy = self.height - 1
self.set_pos_dir(sx, sy, Maze.DOWN, Maze.OPEN)
else:
sy = random.randrange(self.height)
if random.random() < 0.5:
sx = 0
self.set_pos_dir(sx, sy, Maze.LEFT, Maze.OPEN)
else:
sx = self.width - 1
self.set_pos_dir(sx, sy, Maze.RIGHT, Maze.OPEN)
self.start = (sx, sy)
been = [self.start]
pos = -1
solved = False
generate_status = 0
old_generate_status = 0
while len(been) < self.area():
(x, y) = been[pos]
sd = Maze.random_dir()
for nd in range(4):
d = (sd + nd) % 4
if self.get_pos_dir(x, y, d) != Maze.WALL:
continue
(nx, ny) = Maze.go_dir(x, y, d)
if (nx, ny) in been:
continue
if self.is_inside(nx, ny):
self.set_pos_dir(x, y, d, Maze.OPEN)
been.append((nx, ny))
pos = -1
generate_status = len(been) / self.area()
if generate_status - old_generate_status > 0.1:
old_generate_status = generate_status
print(f"{generate_status * 100:.2f}%")
break
elif solved or len(been) < self.min_lenght():
continue
else:
self.set_pos_dir(x, y, d, Maze.OPEN)
self.end = (x, y)
solved = True
pos = -1 - random.randrange(len(been))
break
else:
pos -= 1
if pos < -len(been):
pos = -1
self.key = None
while(self.key == None):
kx = random.randrange(self.width)
ky = random.randrange(self.height)
if (Maze.distance(self.start, (kx,ky)) > self.min_distance()
and Maze.distance(self.end, (kx,ky)) > self.min_distance()):
self.key = (kx, ky)
def get_label(self, x, y):
if (x, y) == self.start:
c = 'S'
elif (x, y) == self.end:
c = 'E'
elif (x, y) == self.key:
c = 'K'
else:
c = ' '
return c
def map(self, moves=[]):
map = ''
for py in range(self.height * 2 + 1):
row = ''
for px in range(self.width * 2 + 1):
x = int(px / 2)
y = int(py / 2)
if py % 2 == 0: #Even rows
if px % 2 == 0:
c = '+'
else:
v = self.get_pos_dir(x, y, self.UP)
if v == Maze.OPEN:
c = ' '
elif v == Maze.WALL:
c = '-'
else: # Odd rows
if px % 2 == 0:
v = self.get_pos_dir(x, y, self.LEFT)
if v == Maze.OPEN:
c = ' '
elif v == Maze.WALL:
c = '|'
else:
c = self.get_label(x, y)
if c == ' ' and [x, y] in moves:
c = '*'
row += c
map += row + 'n'
return map
app = Flask(__name__)
@app.route('/')
def hello_maze():
return "<p>Hello, Maze!</p>"
@app.route('/maze/map', methods=['GET', 'POST'])
def maze_map():
if not ready:
return Response(status=503, retry_after=10)
if request.method == 'GET':
return '<pre>' + maze.map() + '</pre>'
else:
moves = request.get_json()
return maze.map(moves)
@app.route('/maze/start')
def maze_start():
if not ready:
return Response(status=503, retry_after=10)
start = { 'x': maze.start[0], 'y': maze.start[1] }
return json.dumps(start)
@app.route('/maze/size')
def maze_size():
if not ready:
return Response(status=503, retry_after=10)
size = { 'width': maze.width, 'height': maze.height }
return json.dumps(size)
@app.route('/maze/pos/<int:y>/<int:x>')
def maze_pos(y, x):
if not ready:
return Response(status=503, retry_after=10)
pos = {
'here': maze.get_label(x, y),
'up': maze.get_pos_dir(x, y, Maze.UP),
'down': maze.get_pos_dir(x, y, Maze.DOWN),
'left': maze.get_pos_dir(x, y, Maze.LEFT),
'right': maze.get_pos_dir(x, y, Maze.RIGHT),
}
return json.dumps(pos)
WIDTH = 80
HEIGHT = 20
maze = Maze(WIDTH, HEIGHT)
ready = True
The only requirement for the maze model (in requirements.txt
) is the Flask
module.
To create a container image running the maze model, I use this Dockerfile
.
FROM --platform=linux/amd64 public.ecr.aws/docker/library/python:3.12-alpine
WORKDIR /app
COPY requirements.txt requirements.txt
RUN pip3 install -r requirements.txt
COPY . .
CMD [ "python3", "-m" , "flask", "run", "--host=0.0.0.0", "--port=5555"]
Here’s the code for the agent (agent.py
). First, the agent asks the model for the size of the maze and the starting position. Then, it applies its own strategy to explore and solve the maze. In this implementation, the agent chooses its route at random, trying to avoid following the same path more than once.
import random
import requests
from requests.adapters import HTTPAdapter, Retry
HOST = '127.0.0.1'
PORT = 5555
BASE_URL = f"http://{HOST}:{PORT}/maze"
UP, RIGHT, DOWN, LEFT = 0, 1, 2, 3
OPEN, WALL = 0, 1
s = requests.Session()
retries = Retry(total=10,
backoff_factor=1)
s.mount('http://', HTTPAdapter(max_retries=retries))
r = s.get(f"{BASE_URL}/size")
size = r.json()
print('SIZE', size)
r = s.get(f"{BASE_URL}/start")
start = r.json()
print('START', start)
y = start['y']
x = start['x']
found_key = False
been = set((x, y))
moves = [(x, y)]
moves_stack = [(x, y)]
while True:
r = s.get(f"{BASE_URL}/pos/{y}/{x}")
pos = r.json()
if pos['here'] == 'K' and not found_key:
print(f"({x}, {y}) key found")
found_key = True
been = set((x, y))
moves_stack = [(x, y)]
if pos['here'] == 'E' and found_key:
print(f"({x}, {y}) exit")
break
dirs = list(range(4))
random.shuffle(dirs)
for d in dirs:
nx, ny = x, y
if d == UP and pos['up'] == 0:
ny -= 1
if d == RIGHT and pos['right'] == 0:
nx += 1
if d == DOWN and pos['down'] == 0:
ny += 1
if d == LEFT and pos['left'] == 0:
nx -= 1
if nx < 0 or nx >= size['width'] or ny < 0 or ny >= size['height']:
continue
if (nx, ny) in been:
continue
x, y = nx, ny
been.add((x, y))
moves.append((x, y))
moves_stack.append((x, y))
break
else:
if len(moves_stack) > 0:
x, y = moves_stack.pop()
else:
print("No moves left")
break
print(f"Solution length: {len(moves)}")
print(moves)
r = s.post(f'{BASE_URL}/map', json=moves)
print(r.text)
s.close()
The only dependency of the agent (in requirements.txt
) is the requests
module.
This is the Dockerfile
I use to create a container image for the agent.
FROM --platform=linux/amd64 public.ecr.aws/docker/library/python:3.12-alpine
WORKDIR /app
COPY requirements.txt requirements.txt
RUN pip3 install -r requirements.txt
COPY . .
CMD [ "python3", "agent.py"]
You can easily run this simplified version of a simulation locally, but the cloud allows you to run it at larger scale (for example, with a much bigger and more detailed maze) and to test multiple agents to find the best strategy to use. In a real-world scenario, the improvements to the agent would then be implemented into a physical device such as a self-driving car or a robot vacuum cleaner.
Running a simulation using multi-container jobs
To run a job with AWS Batch, I need to configure three resources:
- The compute environment in which to run the job
- The job queue in which to submit the job
- The job definition describing how to run the job, including the container images to use
In the AWS Batch console, I choose Compute environments from the navigation pane and then Create. Now, I have the choice of using Fargate, Amazon EC2, or Amazon EKS. Fargate allows me to closely match the resource requirements that I specify in the job definitions. However, simulations usually require access to a large but static amount of resources and use GPUs to accelerate computations. For this reason, I select Amazon EC2.
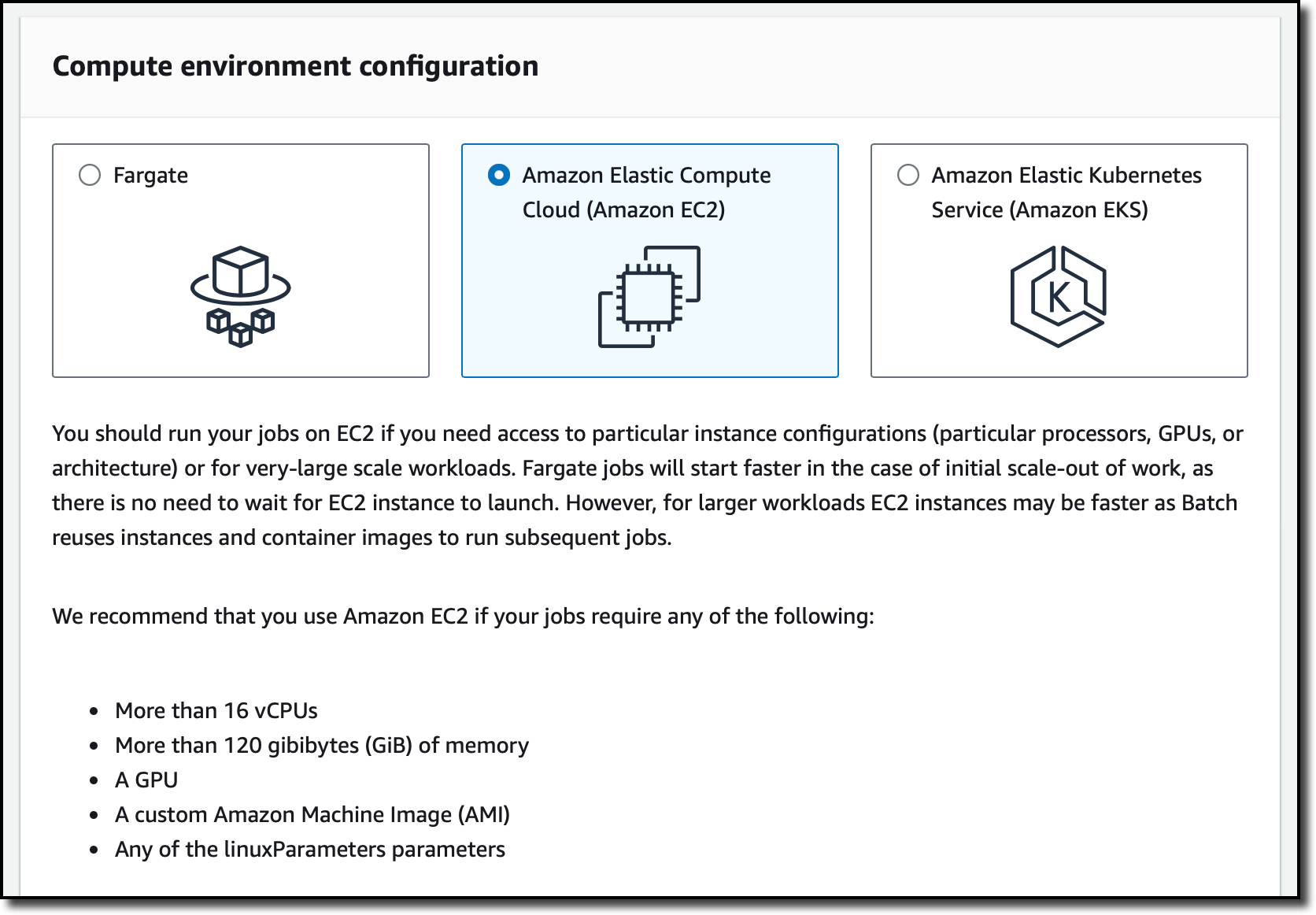
I select the Managed orchestration type so that AWS Batch can scale and configure the EC2 instances for me. Then, I enter a name for the compute environment and select the service-linked role (that AWS Batch created for me previously) and the instance role that is used by the ECS container agent (running on the EC2 instances) to make calls to the AWS API on my behalf. I choose Next.
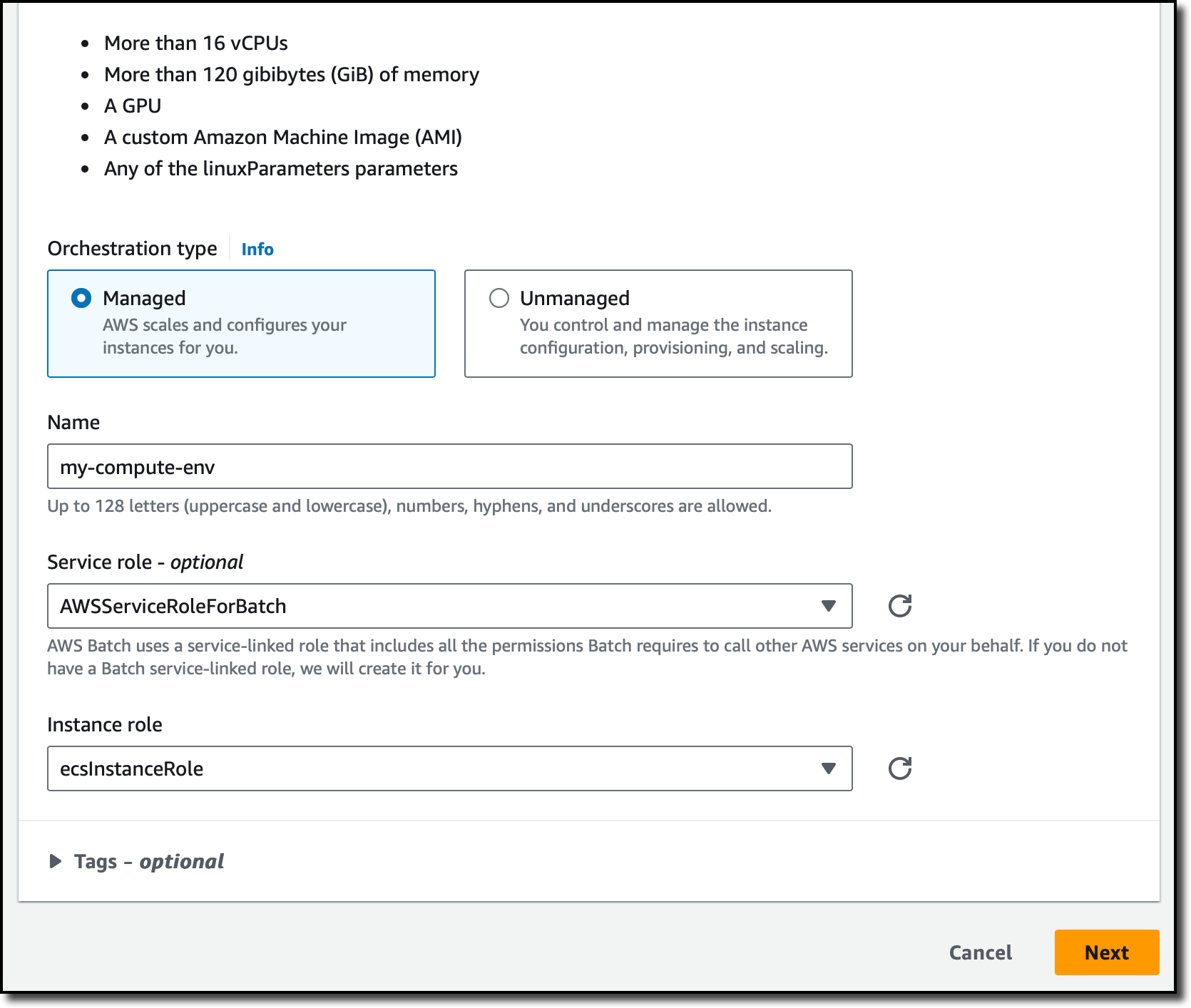
In the Instance configuration settings, I choose the size and type of the EC2 instances. For example, I can select instance types that have GPUs or use the Graviton processor. I do not have specific requirements and leave all the settings to their default values. For Network configuration, the console already selected my default VPC and the default security group. In the final step, I review all configurations and complete the creation of the compute environment.
Now, I choose Job queues from the navigation pane and then Create. Then, I select the same orchestration type I used for the compute environment (Amazon EC2). In the Job queue configuration, I enter a name for the job queue. In the Connected compute environments dropdown, I select the compute environment I just created and complete the creation of the queue.
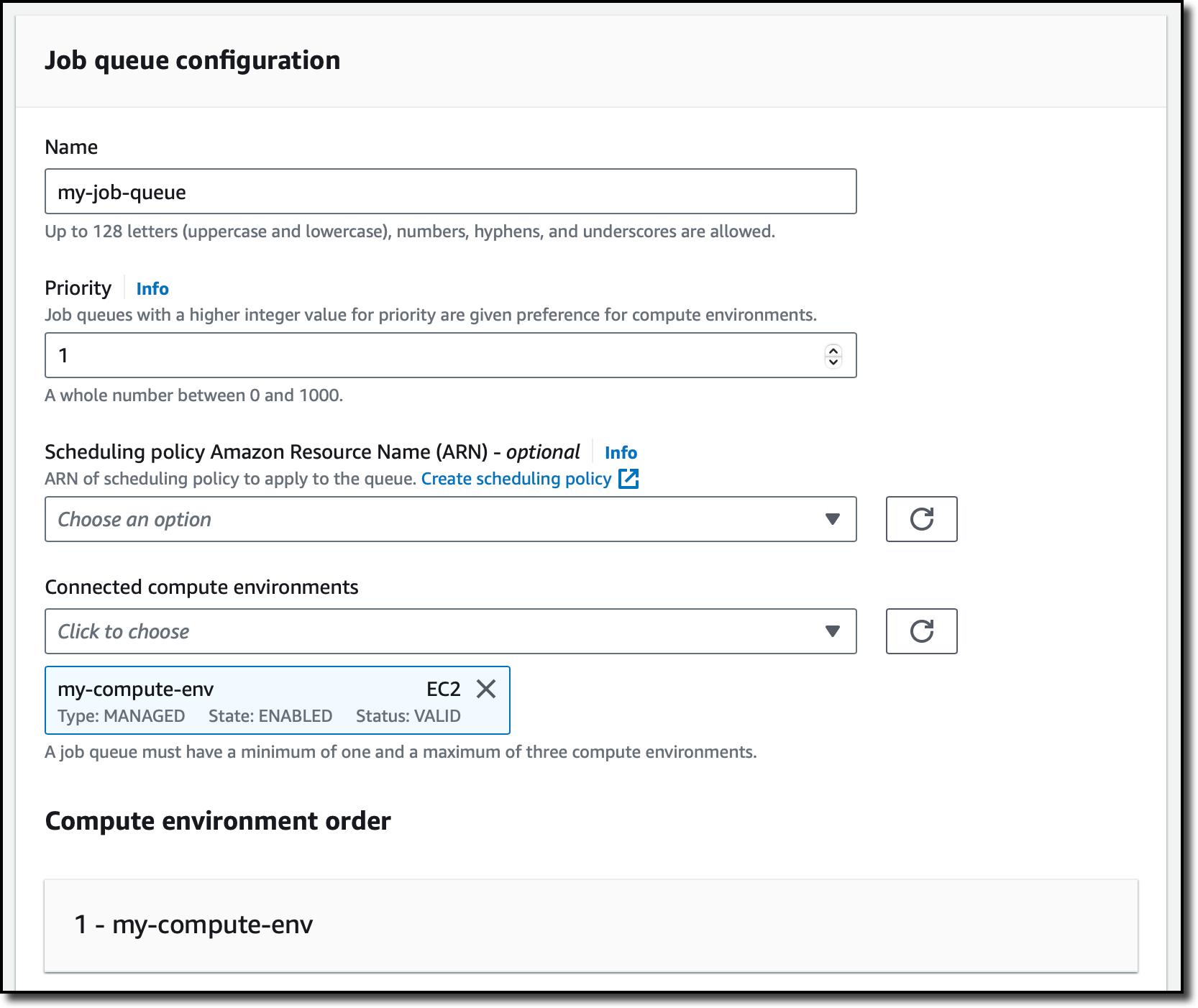
I choose Job definitions from the navigation pane and then Create. As before, I select Amazon EC2 for the orchestration type.
To use more than one container, I disable the Use legacy containerProperties structure option and move to the next step. By default, the console creates a legacy single-container job definition if there’s already a legacy job definition in the account. That’s my case. For accounts without legacy job definitions, the console has this option disabled.
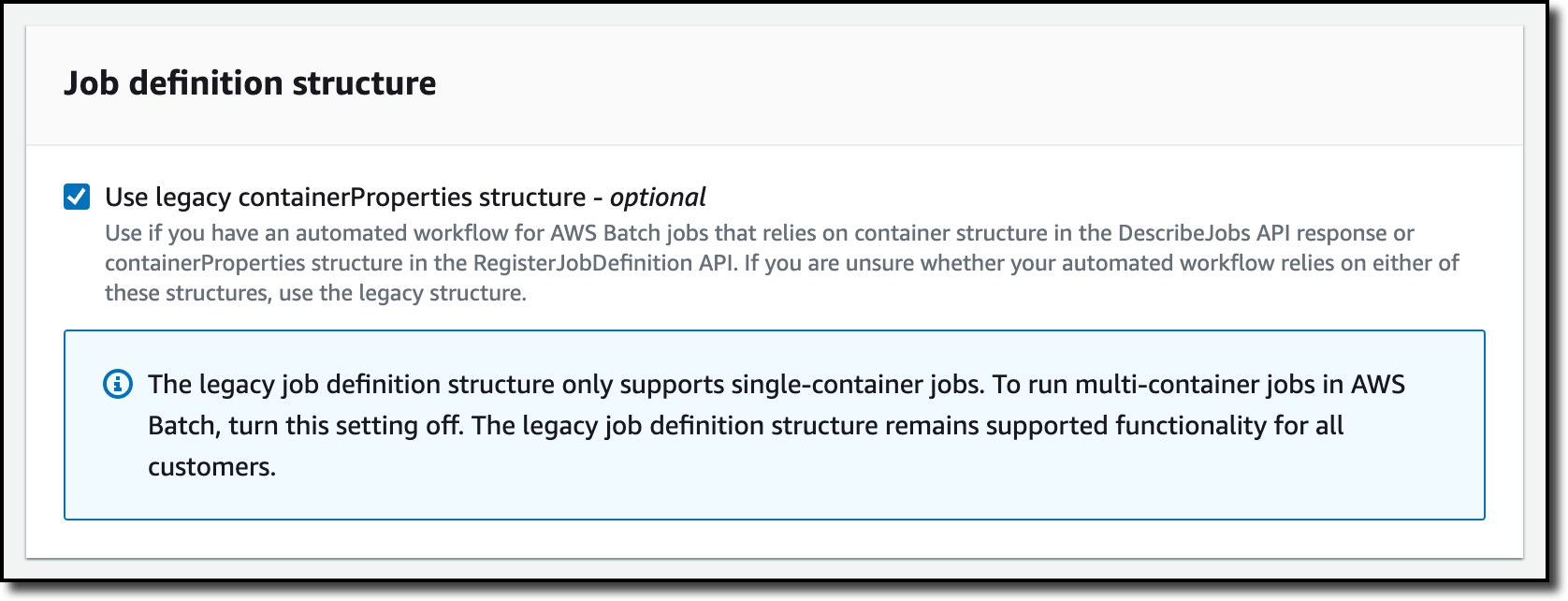
I enter a name for the job definition. Then, I have to think about which permissions this job requires. The container images I want to use for this job are stored in Amazon ECR private repositories. To allow AWS Batch to download these images to the compute environment, in the Task properties section, I select an Execution role that gives read-only access to the ECR repositories. I don’t need to configure a Task role because the simulation code is not calling AWS APIs. For example, if my code was uploading results to an Amazon Simple Storage Service (Amazon S3) bucket, I could select here a role giving permissions to do so.
In the next step, I configure the two containers used by this job. The first one is the maze-model
. I enter the name and the image location. Here, I can specify the resource requirements of the container in terms of vCPUs, memory, and GPUs. This is similar to configuring containers for an ECS task.
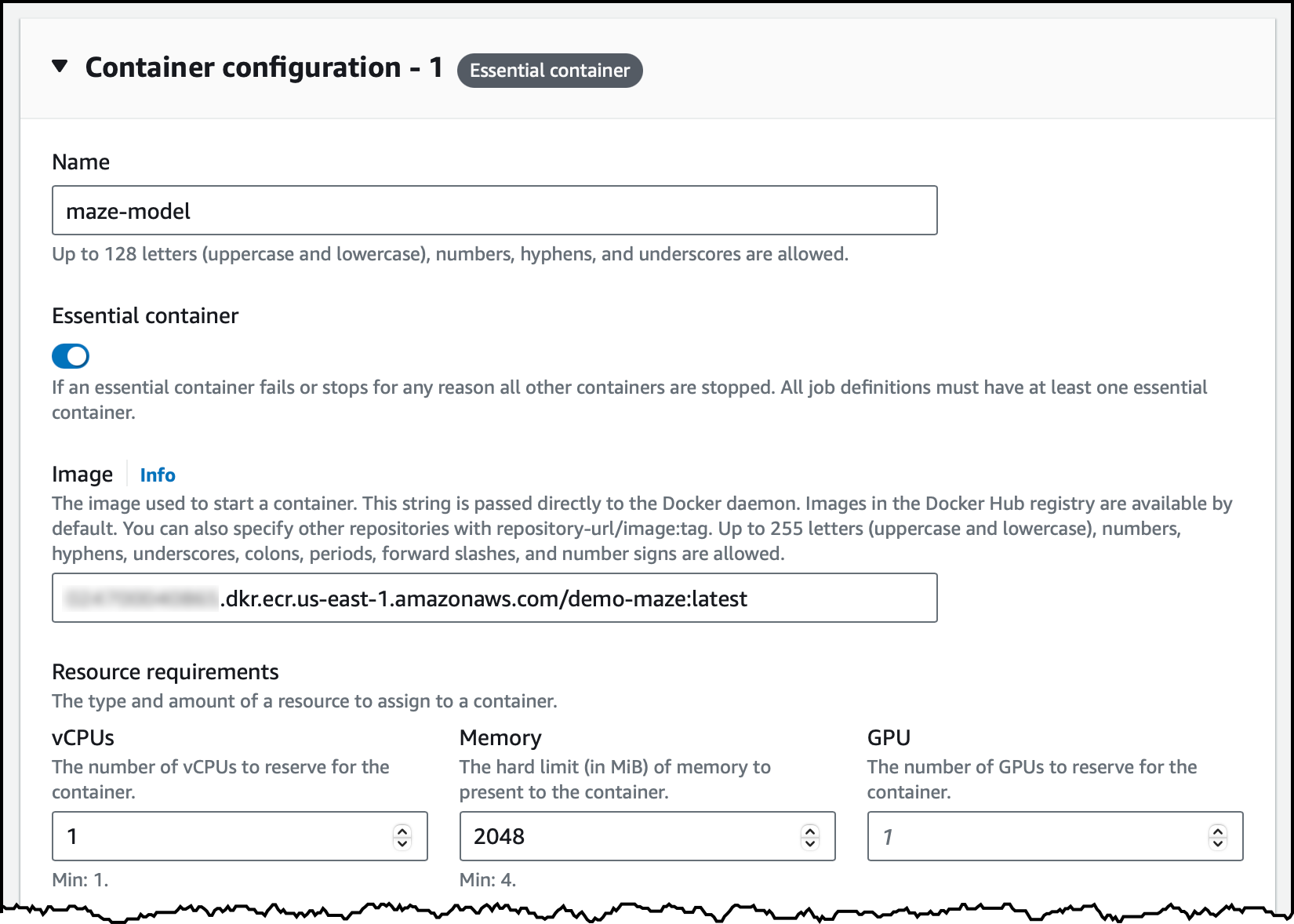
I add a second container for the agent and enter name, image location, and resource requirements as before. Because the agent needs to access the maze as soon as it starts, I use the Dependencies section to add a container dependency. I select maze-model
for the container name and START as the condition. If I don’t add this dependency, the agent
container can fail before the maze-model
container is running and able to respond. Because both containers are flagged as essential in this job definition, the overall job would terminate with a failure.
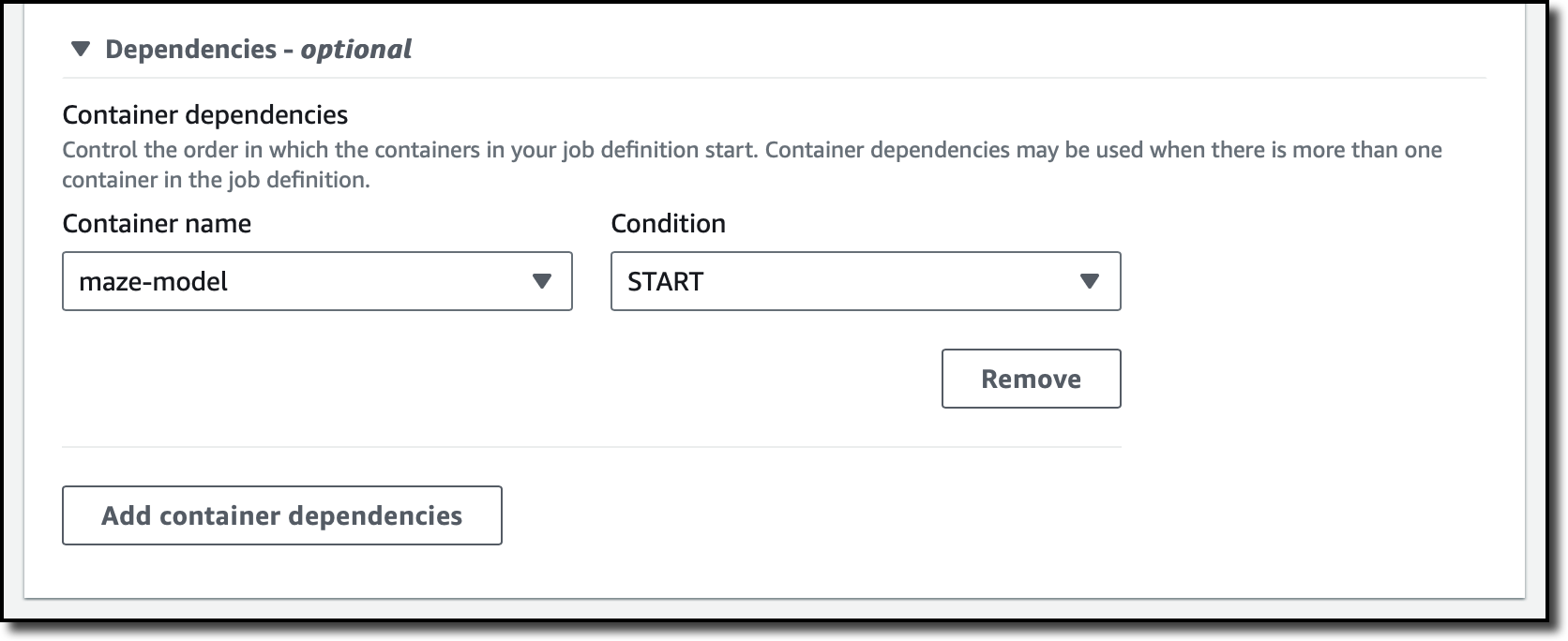
I review all configurations and complete the job definition. Now, I can start a job.
In the Jobs section of the navigation pane, I submit a new job. I enter a name and select the job queue and the job definition I just created.
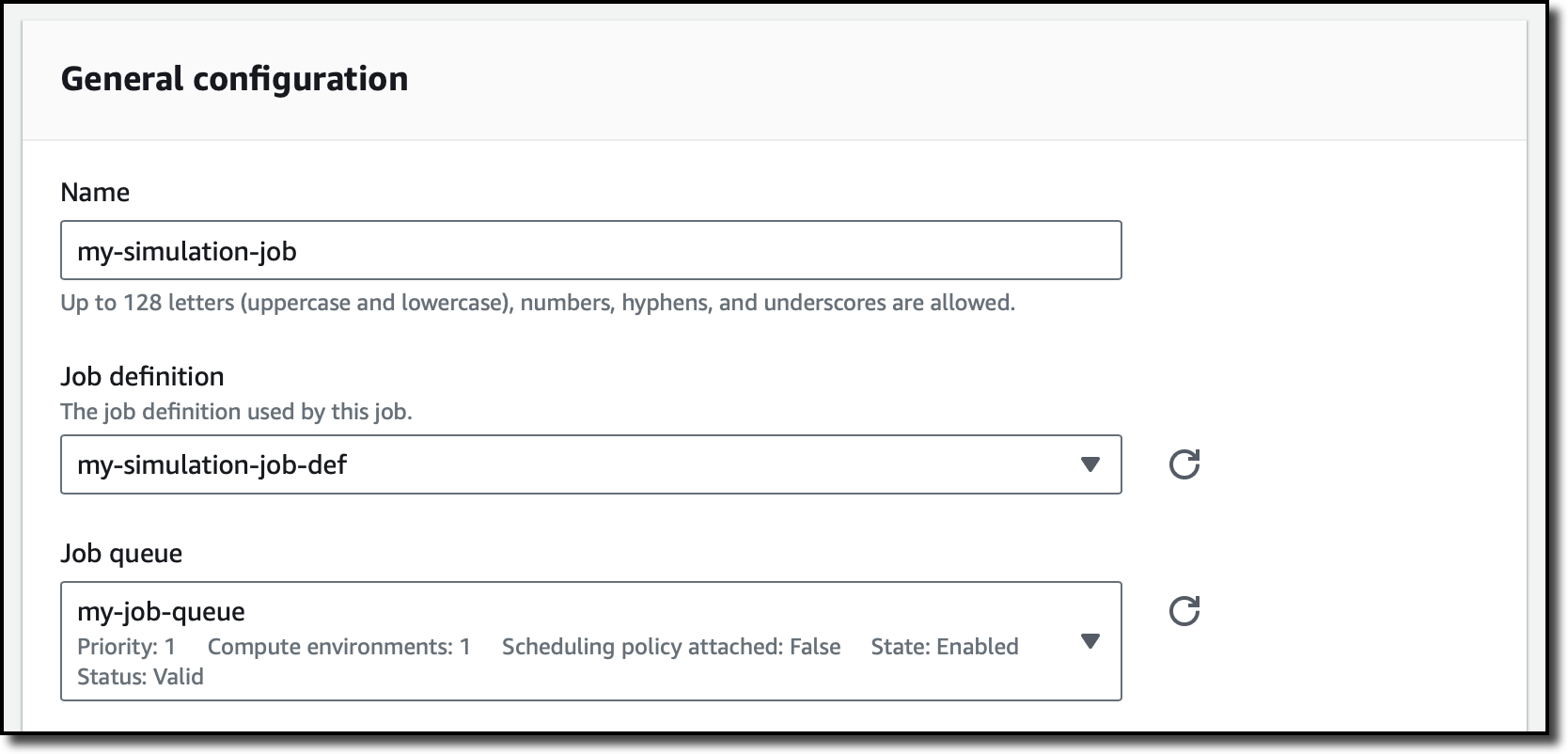
In the next steps, I don’t need to override any configuration and create the job. After a few minutes, the job has succeeded, and I have access to the logs of the two containers.
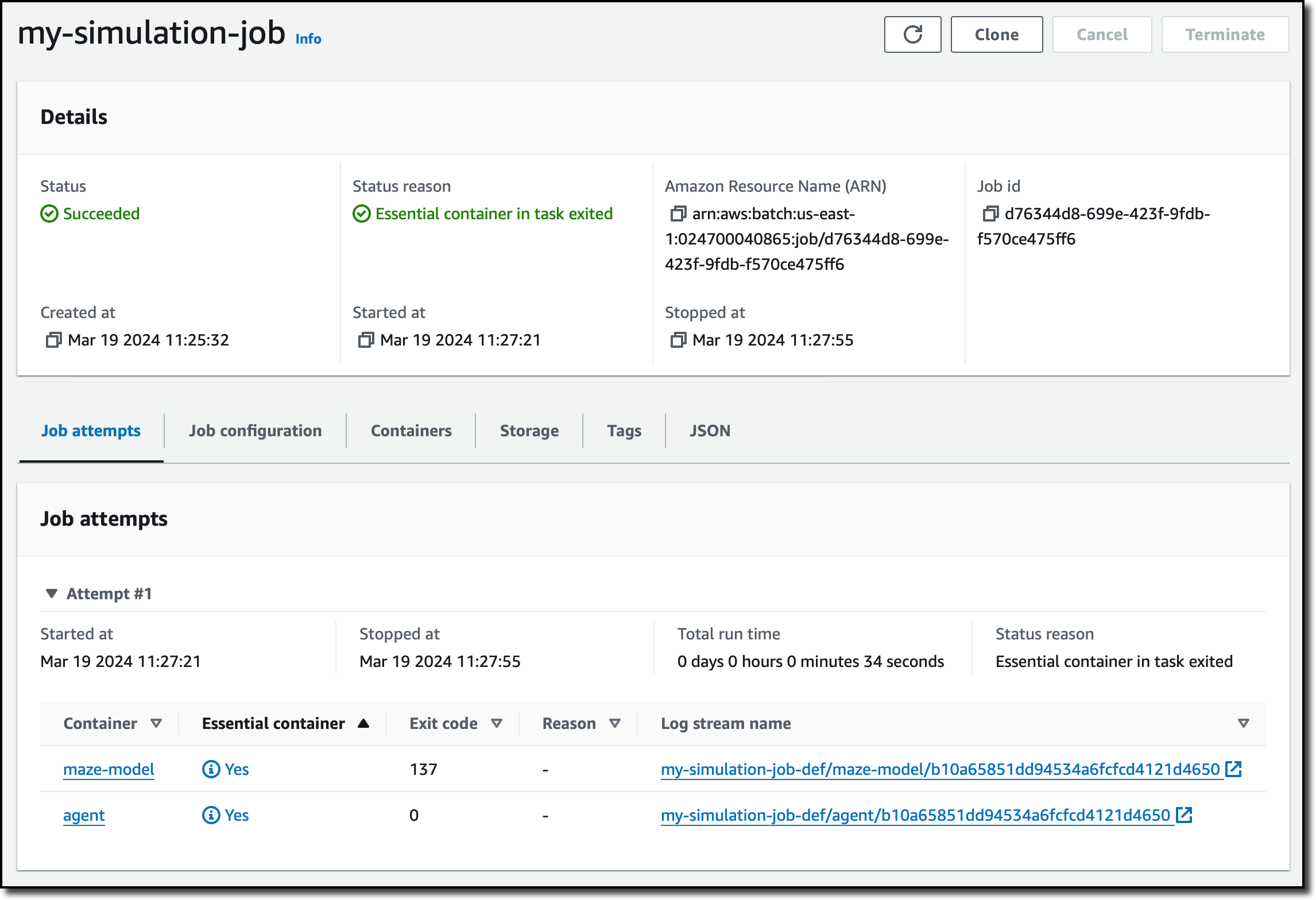
The agent solved the maze, and I can get all the details from the logs. Here’s the output of the job to see how the agent started, picked up the key, and then found the exit.
SIZE {'width': 80, 'height': 20}
START {'x': 0, 'y': 18}
(32, 2) key found
(79, 16) exit
Solution length: 437
[(0, 18), (1, 18), (0, 18), ..., (79, 14), (79, 15), (79, 16)]
In the map, the red asterisks (*) follow the path used by the agent between the start (S), key (K), and exit (E) locations.
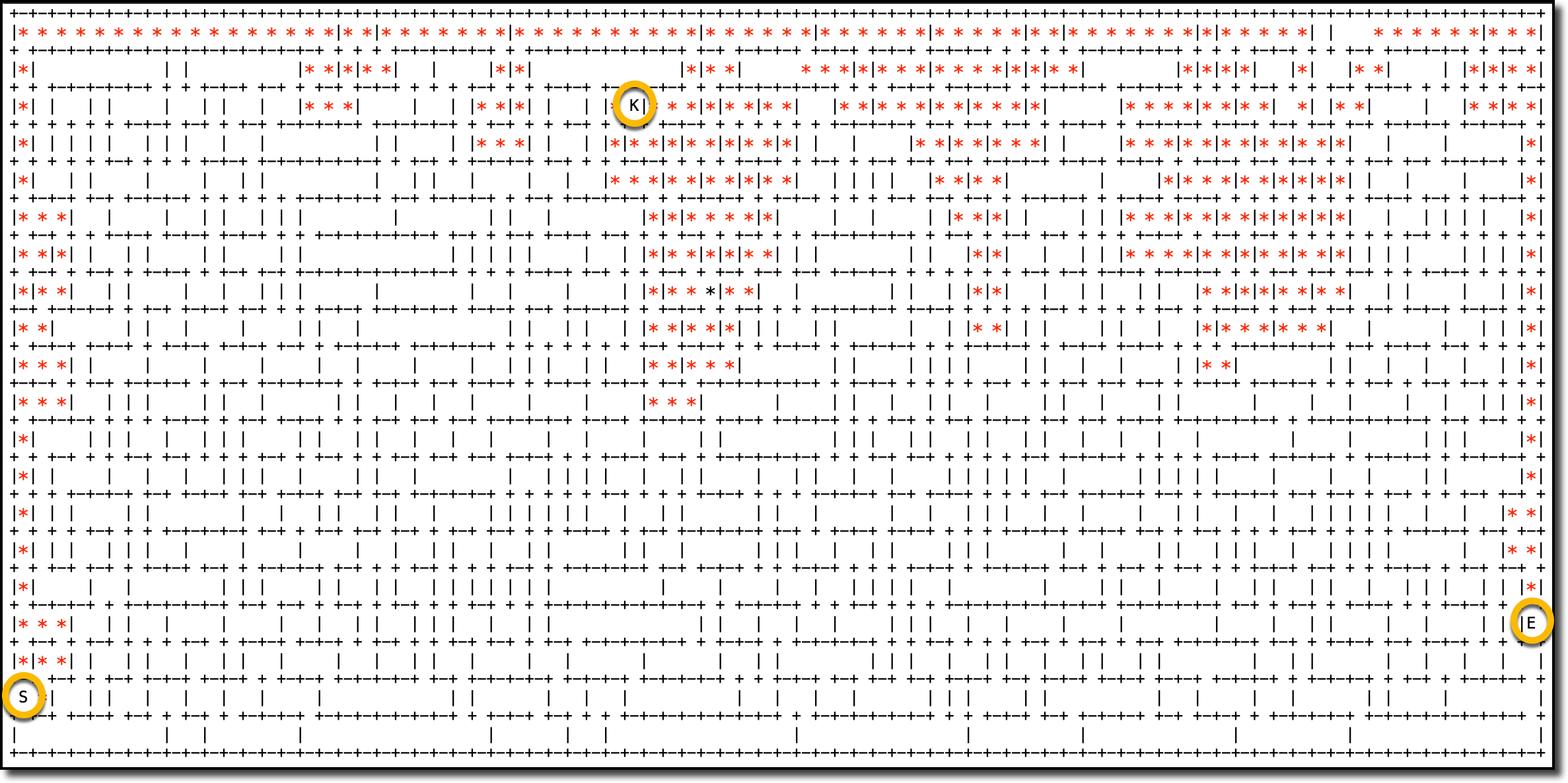
Increasing observability with a sidecar container
When running complex jobs using multiple components, it helps to have more visibility into what these components are doing. For example, if there is an error or a performance problem, this information can help you find where and what the issue is.
To instrument my application, I use AWS Distro for OpenTelemetry:
Using telemetry data collected in this way, I can set up dashboards (for example, using CloudWatch or Amazon Managed Grafana) and alarms (with CloudWatch or Prometheus) that help me better understand what is happening and reduce the time to solve an issue. More generally, a sidecar container can help integrate telemetry data from AWS Batch jobs with your monitoring and observability platforms.
Things to know
AWS Batch support for multi-container jobs is available today in the AWS Management Console, AWS Command Line Interface (AWS CLI), and AWS SDKs in all AWS Regions where Batch is offered. For more information, see the AWS Services by Region list.
There is no additional cost for using multi-container jobs with AWS Batch. In fact, there is no additional charge for using AWS Batch. You only pay for the AWS resources you create to store and run your application, such as EC2 instances and Fargate containers. To optimize your costs, you can use Reserved Instances, Savings Plan, EC2 Spot Instances, and Fargate in your compute environments.
Using multi-container jobs accelerates development times by reducing job preparation efforts and eliminates the need for custom tooling to merge the work of multiple teams into a single container. It also simplifies DevOps by defining clear component responsibilities so that teams can quickly identify and fix issues in their own areas of expertise without distraction.
To learn more, see how to set up multi-container jobs in the AWS Batch User Guide.
— Danilo